The Power of Machine Learning Labeling Tools in Business
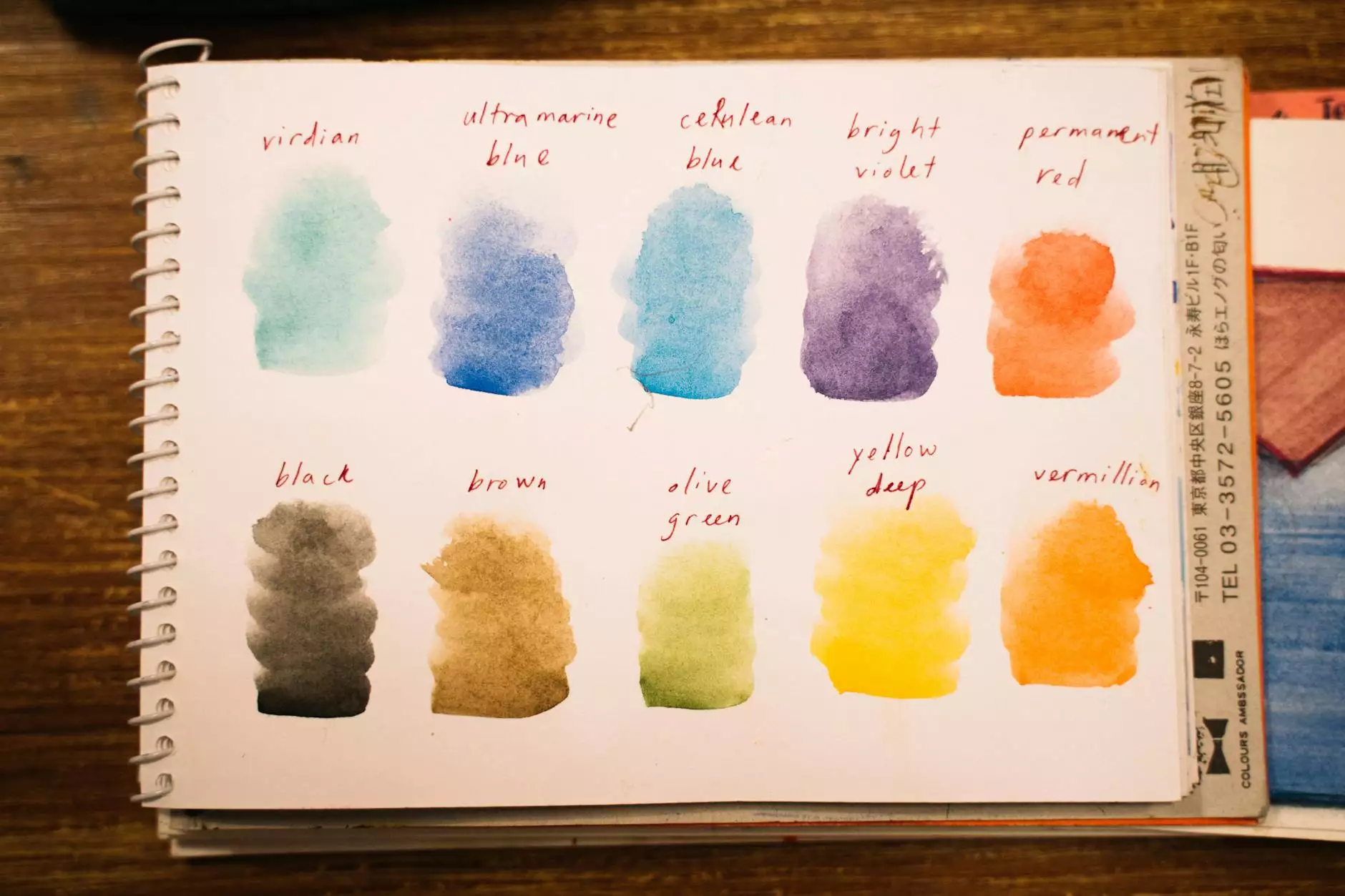
In today’s rapidly evolving technology landscape, businesses are seeking ways to leverage data for growth and efficiency. One of the most critical components in harnessing the potential of data is through effective data annotation. As organizations aim to develop AI and machine learning models, the demand for high-quality labeled data skyrockets. This is where machine learning labeling tools step in, revolutionizing the way data is processed, analyzed, and utilized.
Understanding the Importance of Data Annotation
Before delving into the specifics of machine learning labeling tools, it’s essential to understand data annotation and why it is pivotal for machine learning projects. Data annotation refers to the process of labeling data inputs, including images, videos, and text, enabling algorithms to learn from them.
Effective data annotation can significantly enhance model accuracy and performance. When data is correctly annotated, it provides a reliable foundation for machine learning models to identify patterns, make predictions, and execute tasks autonomously. Thus, the quality of the annotations directly impacts the effectiveness of the resulting AI solutions.
What is a Machine Learning Labeling Tool?
A machine learning labeling tool is a software solution designed to facilitate the data annotation process. These tools provide businesses with the means to efficiently and accurately tag their datasets. The significance of these tools includes:
- Automated Annotation: Many tools offer automation features that drastically reduce the time required for labeling.
- Collaboration: Teams can work together in real-time, sharing insights and feedback to improve data quality.
- Scalability: Tools can scale to handle large datasets, which is essential for modern enterprises.
- User-Friendly Interfaces: Most tools feature intuitive designs that lower the barrier for users, allowing non-technical staff to participate in data annotation.
Key Features of Effective Machine Learning Labeling Tools
The effectiveness of a machine learning labeling tool can be assessed based on several critical features:
1. Versatile Annotation Capabilities
Good labeling tools support various data types, including text, images, audio, and video. This versatility enables businesses to annotate diverse datasets efficiently.
2. Support for Machine Learning Frameworks
Integration capabilities with popular machine learning frameworks (like TensorFlow, PyTorch, etc.) ensure seamless workflows, making it easier to transition from data labeling to model training.
3. Quality Control Mechanisms
Advanced quality control features, including validation checkpoints and review stages, help maintain high data quality. This is crucial as even a small percentage of incorrect annotations can lead to poor model performance.
4. Customization Options
The ability to create custom workflows tailored to specific organizational needs enhances the efficiency of the annotation process.
5. Data Security and Compliance
Data privacy is paramount in today’s business operations. Reputable machine learning labeling tools implement robust security measures to protect sensitive data, ensuring compliance with regulations like GDPR.
Benefits of Integrating Machine Learning Labeling Tools in Business
The integration of machine learning labeling tools within business frameworks presents multiple benefits that can lead to significant competitive advantages:
- Enhanced Productivity: Automated features and collaborative tools significantly reduce the time spent on data annotation, allowing teams to focus on higher-value tasks.
- Improved Accuracy: With the combination of automated and manual quality controls, organizations can achieve higher levels of accuracy in their labeled datasets.
- Faster Time to Market: Streamlined annotation processes allow businesses to speed up the deployment of machine learning models, leading to faster product rollouts.
- Cost Efficiency: Reducing the resource allocation for data labeling can lead to significant cost savings over time.
- Better Decision Making: High-quality labeled data leads to better machine learning models, which in turn improve decision-making processes across all levels of an organization.
Case Studies: Success Stories with Machine Learning Labeling Tools
1. Retail Industry Case Study
One prominent retail company implemented a machine learning labeling tool to annotate customer feedback data. By organizing and analyzing this data more effectively, they were able to identify trends and preferences, allowing for better inventory management and targeted marketing strategies that led to a 20% increase in sales.
2. Healthcare Sector Innovation
A healthcare provider utilized a labeling tool to annotate medical images used for diagnostic purposes. Through precise labeling, they enhanced the predictive capabilities of their machine learning algorithms, which resulted in earlier detection of diseases and improved patient outcomes.
Choosing the Right Machine Learning Labeling Tool
With numerous machine learning labeling tools available today, selecting the right one can be daunting. Here are key considerations when choosing a tool:
- Compatibility: Ensure that the tool integrates well with existing data science workflows and technologies.
- Customer Support: Adequate support and training resources can facilitate smoother implementation and ongoing use.
- Pricing Structure: Analyze the pricing model to ensure it aligns with your budget and offers good value for the features provided.
- User Reviews: Look for testimonials and case studies from other businesses in your industry to gauge effectiveness and satisfaction.
The Future of Machine Learning Labeling Tools
As artificial intelligence continues to advance, machine learning labeling tools are expected to evolve as well. Here are some anticipated trends:
- Increased Automation: The rise of AI-driven automation will likely create labeling tools that can learn and adapt over time, improving accuracy continuously.
- Enhanced User Experience: As competition grows, providers will focus on making their tools more intuitive and user-friendly.
- Rich Analytics Features: Future tools may incorporate advanced analytics that provide insights into the efficiency of labeling processes and data quality.
Conclusion
The increasing reliance on data-driven strategies in business underscores the necessity for effective data annotation tools. Machine learning labeling tools stand at the forefront, enabling organizations to annotate large datasets quickly and accurately, leading to better AI and machine learning outcomes. By embracing these tools, businesses not only enhance productivity and reduce costs but also position themselves for long-term success in a data-centric world.
Explore how KeyLabs.ai can equip your organization with leading data annotation tools to maximize your machine learning endeavors today!